Machine learning (ML) is one of the most transformative technologies of the 21st century. With its ability to enable machines to learn from data and improve over time, it is revolutionizing industries and shaping the future of technology. From healthcare and finance to entertainment and autonomous vehicles, this technology is making a significant impact. In this article, we will explore the basics of ML, its applications, types, and the future of this groundbreaking technology.
What is Machine Learning?
At its core, machine learning is a subset of artificial intelligence (AI) that focuses on developing algorithms that allow computers to learn and make predictions from data. These algorithms enable systems to recognize patterns and improve their predictions without being explicitly programmed.
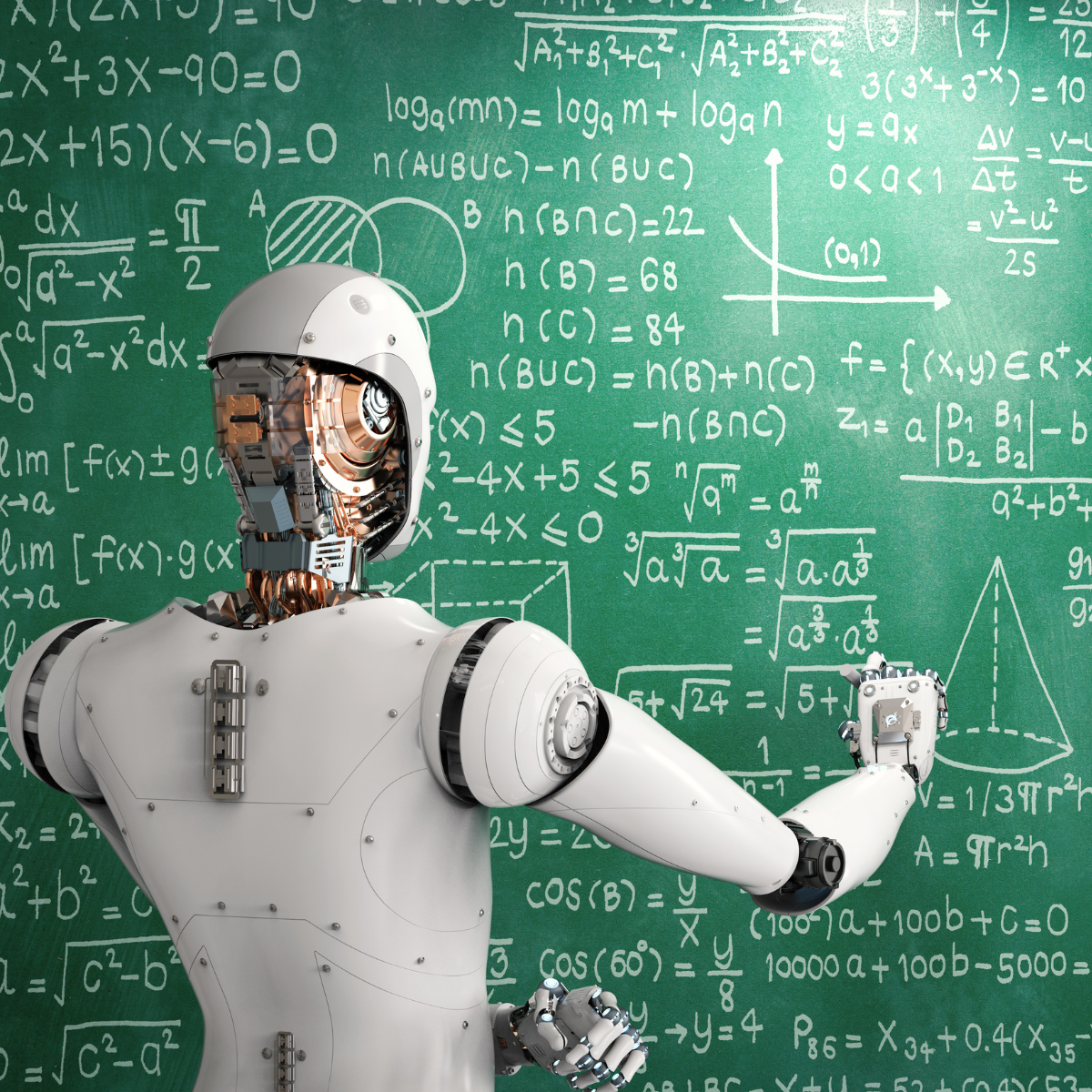
Machine learning enables systems to learn from data, improving predictions and decision-making processes.
The technology relies on feeding machines with data, enabling them to process and recognize trends without human intervention. As they are exposed to more data, the systems become better at predicting outcomes and improving their accuracy.
How Does ML Work?
Several key components drive the process:
- Data: The foundation of machine learning is data. High-quality datasets are essential to train the algorithms effectively. Diverse and comprehensive data improves the machine’s ability to perform complex tasks.
- Algorithms: These are the mathematical models used to analyze data. They enable the system to detect patterns and refine its predictions based on learned experiences.
- Training: During training, the algorithm is exposed to data and adjusts its internal parameters to improve its accuracy.
- Testing: After training, the system is tested with new, unseen data to assess its predictive abilities. This helps evaluate the model’s performance and reliability.
- Prediction: Once trained and tested, the model can make predictions based on fresh data inputs.
Types of Machine Learning
Machine learning can be classified into three main types: supervised learning, unsupervised learning, and reinforcement learning. These approaches are used based on the data available and the specific tasks at hand.
1. Supervised Learning
Supervised learning is the most common approach, where the algorithm is trained on labeled data. In this method, both the input and the corresponding output are provided, allowing the system to learn the relationship between the two.
Applications:
- Spam email filtering: The algorithm can classify emails as spam or not based on labeled examples.
- Speech recognition: Machines learn to identify words and phrases from a dataset of labeled audio.
2. Unsupervised Learning
Unsupervised learning works with unlabeled data. The algorithm tries to find hidden patterns without knowing the desired outcome in advance.
Applications:
- Customer segmentation: Businesses use this method to group customers based on purchasing behavior.
- Anomaly detection: Used to identify unusual behaviors or fraudulent activities.
3. Reinforcement Learning
Reinforcement learning is inspired by behavioral psychology. In this approach, an agent learns to make decisions by interacting with its environment and receiving feedback in the form of rewards or penalties.
Applications:
- Self-driving cars: Reinforcement learning teaches autonomous vehicles how to navigate and make decisions on the road.
- Game AI: Systems like AlphaGo use reinforcement learning to improve their gameplay.
Applications of Machine Learning
This technology is having a profound impact across industries. Let’s look at some key applications:
1. Healthcare
In healthcare, ML is improving diagnostics, personalized treatment, and drug development. Algorithms analyze medical images, lab results, and patient histories to help detect diseases early.
Additionally, ML is used to predict the likelihood of diseases like diabetes and heart conditions, allowing for timely interventions and better patient outcomes.
2. Finance
Financial institutions are employing this technology for fraud detection, risk management, and automated trading. Systems can analyze transaction data in real-time, identifying fraudulent activities.
ML also improves credit scoring and loan approval processes, where models assess an applicant’s financial behavior to predict their creditworthiness.
3. Retail and E-commerce
Retailers and e-commerce platforms leverage ML for product recommendations, inventory optimization, and customer support. By analyzing customer purchase history, ML algorithms can predict future buying behavior and offer personalized product suggestions.
For example, Amazon’s recommendation engine uses these techniques to suggest products based on past browsing or purchasing activity.
4. Autonomous Vehicles
ML is key in the development of autonomous vehicles. By processing data from cameras, radar, and sensors, self-driving cars can navigate and make real-time decisions.
Leading companies like Tesla and Waymo use these technologies to improve the safety and efficiency of self-driving cars, adapting to changing environments.
5. Entertainment and Media
Streaming services such as Netflix and Spotify use machine learning to recommend content based on users’ preferences and behaviors. These recommendations improve as the system gathers more data over time.
Additionally, ML is used in gaming to create adaptive non-playable characters (NPCs) that react to player actions, improving the overall gaming experience.
Challenges in Machine Learning
Despite its potential, machine learning faces several challenges:
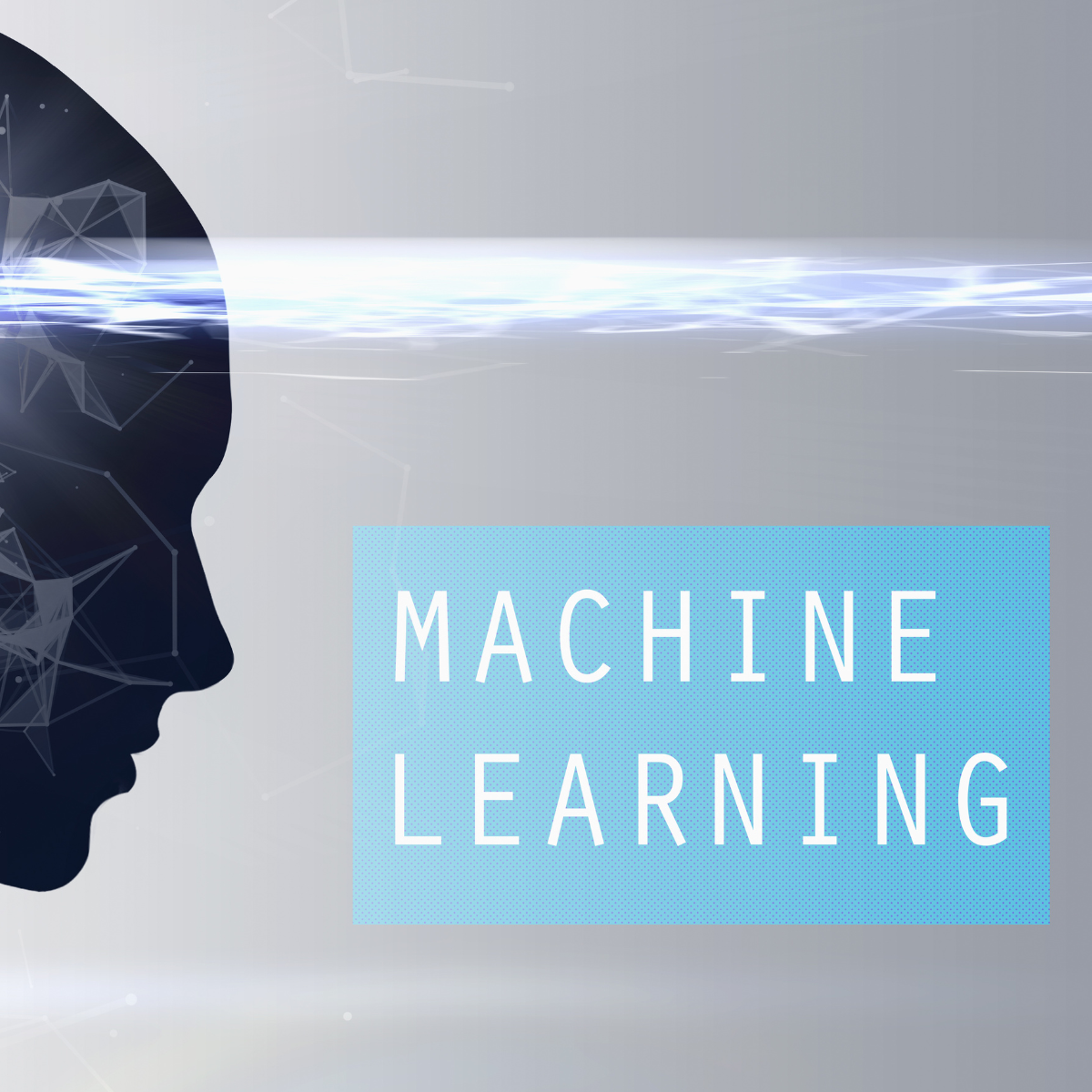
Machine learning is transforming healthcare by enabling early disease detection and personalized treatment plans.
- Data Quality: The effectiveness of ML systems heavily relies on the quality of data. Poor or biased data can lead to inaccurate predictions and skewed results.
- Interpretability: Some ML models, like deep learning, are often considered “black boxes” because their decision-making processes are not easily understood.
- Computational Power: Training complex models requires significant computational resources, which can be time-consuming and expensive.
- Ethical Considerations: Issues surrounding data privacy, algorithmic bias, and accountability are becoming more pressing as the technology advances.
The Future of Machine Learning
As this technology evolves, several trends are shaping its future:
- Explainable AI (XAI): Researchers are working on creating models that provide transparency, making it easier for users to understand how predictions are made.
- Edge Computing: With the growth of IoT, ML will increasingly be deployed closer to devices, reducing reliance on cloud computing.
- AI Ethics and Regulation: As ML becomes more widespread, ethical guidelines and regulations will be crucial to ensure its responsible use.
Conclusion
Machine learning is a powerful technology that is changing how we interact with data and make decisions. From healthcare to autonomous vehicles, its impact is undeniable. While challenges remain, the potential for ML to transform industries is vast. As we move into the future, advancements in AI and machine learning will continue to shape our world in new and exciting ways.
You can read: